Ali-Malek BOUBAYA
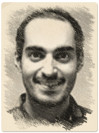
Error estimators, anisotropic meshes, and artificial intelligence coupling for complex industrial multiphase flows.
“The research project deals with the numerical simulation of liquid-vapor interface evolution during the quenching process. Different interface capturing methods (Level-Set, Phase-Field,…) are revisited in order to provide a unified numerical framework for multiphase flows. Robust and efficient solvers are developped within the finite element framework CIMLIB_CFD. These solvers are improved by new stabilization techniques, mesh adaptation, and machine learning.”
Junfeng CHEN
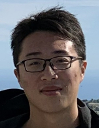
Coupling HPC and Artificial Intelligence for a new turbulence model.
“The aim of this PhD thesis is to develop, test and validate novel modeling approaches where the turbulent model is trained against large data sets of 3D CFD simulations. The objectives are to: (i) create a database of turbulent flows around typical obstacles; (ii) elaborate efficient learning strategies and validate them in unlearned cases; (iii) apply this approach to realistic turbulent flows around complex environment and obtain high-quality monitoring.“
Hassan GHRAIEB
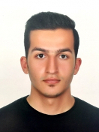
Numerical simulations and artificial intelligence coupling for shape optimization.
“Deep reinforcement learning (DRL) is a new tool recently used in fluid mechanics for decision-making problems (optimization, optimal control, etc…) to maximize a scalar reward signal. The goal of the thesis is to apply DRL algorithms to solve shape optimization problems where the reward is obtained from numerical simulations.”
Ramy NEMER
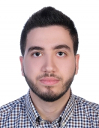
Fluid—Membrane Interaction (FMI) simulation of multiphase complex flows inside a pressure regulator for diving.
“The goal is to optimize and enhance the performance of a pressure regulator for diving, thru numerical simulations. This is done using a hybrid Fluid—Membrane Interaction scheme, which combines the conventional monolithic and partitioned approaches. Furthermore, the outlet boundary condition is of interest, to be able to simulate the highly turbulent flow in our truncated domain.”