Sacha EL AOUAD
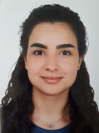
Numerical and parallel modelling of the fluid-solid interaction for heat treatment and the quenching process.
“This thesis focuses on studying the fluid-solid interaction during heat transfer. The aim is to model the temperature gradient on the interface using anisotropic mesh adaptation to be able to compute the thermodynamics properties of each material.”
Joe KHALIL

Modeling fluid solid coupling with residual and thermoelastoplastic stresses analysis and optimization.
“The main objective of the thesis, is to understand the behavior of the materials during quenching. Phase transformation phenomenon occurs during this process, because of the significant temperature change. Both thermal behavior, and phase transformation has an effect on the mechanical response of the materials. The project consists of coupling between the thermal, mechanical and phase transformation effect on the material during quenching, taking into account the change in the thermal properties. In addition, the quenchant (fluid) behavior at the interface with the material, affects the thermal behavior noting that the liquid at the interface passes through different phases which affects the heat flux.”
Ghaniyya MEDGHOUL
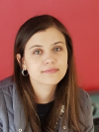
Immersed solid-fluid interfaces in industrial quenching processes in a massively parallel environment with anisotropic meshing.
“The accuracy of a simulation is closely connected to the design of the fluid-solid mesh, whereas the reliability of a simulation is related to the sensitivity of the numerical approximations and degree of discretisation to model parameters and data. Therefore, the general framework of this PhD will mainly tackle on remeshing for which adequate arror estimators need to be derived and dynamic load balancing that take into account rapid changes in the solution and the localized interfaces. A sharp interface approach is considered to cope with the fluid-solid coupling using an energy node-transport equation to ensure interface exactness. This strategy allows the movement of solids inside quenching devices while maintaining high accuracy.”
Aakash PATIL
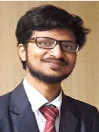
Physics-Informed Deep Learning for Subgrid-Scale Modeling of Turbulent Flows.
“The proposed research deals with the application of Deep Learning (DL) in the context of Large Eddy Simulations (LES). The proposed strategy consists, on the one hand, to detect new physical constraints for model eligibility, and on the other hand, to generate physics-informed local convolutional filters to model the small scales. This will lead to the design of innovative closure strategies, without any a priori on the discretization operators nor on the relevant physical quantities. The data sets used for learning and testing correspond to the state of the art and come from very-high-resolution direct numerical simulations of developed turbulent flows.”