Ricardo EL KHOURY

Biopolymer-induced drag reduction: a promising technique to minimize water and electricity wastes in water pumping systems
The purpose of this project is to reduce both water and electricity wastes in water pumping systems by benefiting from a non-Newtonian fluid mechanics phenomenon called biopolymer drag reduction: a dramatic pressure drop decrease in turbulent flows induced by the addition of a tiny amount of biopolymers. The aloe vera produces a stable and non-toxic natural mucilage that can interact with turbulent flows, partially suppressing their chaotic nature and, consequently, the wall shear stress in pipelines. As a result, a tremendous reduction of pumping costs and water leaks is observed, which ultimately can be converted into productivity gain in farms and/or industrial complexes. The objective is to highlight the physical mechanism driving the biopolymer/flow interactions, narrowing the gap between the fundamental understanding of the turbulent drag reduction phenomenon and quantitative predictions for agricultural and industrial applications. This project is made feasible through a mixed fluid mechanics approach combining experiments, direct numerical simulations, and artificial intelligence.
Paul GARNIER
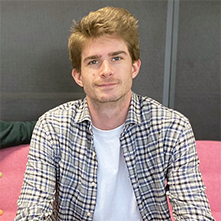
MACHINE LEARNING FOR PERSONALIZED ANEURYSM TREATMENT
We explore the integration of machine learning techniques, including CNNs, transformers, and graph neural networks (GNNs), with computational fluid dynamics (CFD) for simulating and treating intracranial aneurysms (IAs). Pretraining and finetuning strategies in GNNs show promise for training models efficiently, with unsupervised techniques like node masking and could be apply to difficult use-cases such as IAs. Additionally, temporal training methods, such as using transformer architectures for handling temporal data, present opportunities to enhance IA simulations. Future research directions include applying pretraining methodologies to GNNs, addressing challenges with large mesh datasets, and incorporating CFD-specific techniques to further improve IA management.
Vincent LANNELONGUE
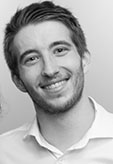
Computational fluid mechanics, 4D flow MRI and deep learning for intracranial aneurysm rupture risk stratification
An intracranial aneurysm is a deformation of a blood vessel wall inside the brain. All intracranial aneurysms do not tear, but a rupture leads to a highly mortal subarachnoid hemorrhage. Several treatments such as surgery or stents exist to prevent such tear of the blood vessel wall, but they carry their own risks of complications. The assessment of the risk of rupture of an aneurysm is key to decide whether or not a patient should undergo treatment or surgery. Recent progress in medical imaging has allowed us to better detect intracranial aneurysms and to establish better 3D models which are a key component in fluid mechanical simulations that can provide a more profound understanding of hemodynamics inside an aneurysm. Moreover, the latest MRI techniques give information on the flow dynamics inside the blood vessels. This work aims at exploiting this new source of data, along with deep learning models, to overcome current limitations in fluid dynamics simulations of the hemodynamics inside the brain arteries, to better evaluate the risk of rupture of intracranial aneurysms.
Tianchi LI
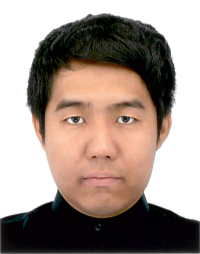
New insights in the reduced mobility description for the modeling of grain growth and recrystallization at the polycrystalline scale
The metallurgical industry, as one of the major carbon dioxide producers in the world, is critical to the success of Net-Zero Transition. In this context, advanced tools for computational metallurgy are in great demand to allow the traditional industry to benefit from the 21th century’s skyrocketing computing powers to improve the design and manufacturing of metallic materials. Knowing that the properties of metallic materials depend heavily on their underlying microstructures, it is extremely important to be able to predict, with a high level of accuracy, their microstructure evolutions, e.g., grain boundary (GB) migration, during thermo-mechanical treatments. This PhD is dedicated to developing state-of-the-art numerical methods for the modeling of GB dynamics during material forming processes. The research work focuses on the enrichment of existing full-field front-capturing and front-tracking methods to integrate some new capabilities such as the use of a tensorial reduced mobility description, a better integration of torque terms in 2D and 3D and a disconnection-based description of the involved mechanisms.
Xuan Minh Vuong NGUYEN

Coupling of Deep Reinforcement Learning with Convolution Graph Neural Networks, for the simulation and the optimization of flows
This doctoral thesis investigates the amalgamation of Deep Reinforcement Learning (DRL) with Graph Neural Networks (GNNs) or Transformers to address the intricacies inherent in simulating and optimizing complex flow systems. A novel framework is proposed, leveraging the spatial perception capabilities of GNNs or Transformers alongside the decision-making prowess of DRL to adeptly model fluid meshes and optimize their configurations. Additionally, the thesis develops various aspects of the proposed framework, including network architecture, training procedures, and simulation environment. Extensive experiments will be conducted to validate the efficacy and scalability of the proposed methodology across diverse flow scenarios. Ultimately, the thesis contributes to enhancing the efficiency and autonomy of fluid mesh optimization systems, thereby holding significance for engineering and scientific applications..
Ugo PELISSIER
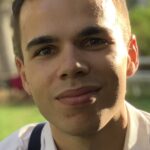
Haemodynamics and thrombus formation for arterial wall remodeling prediction in intracranial aneurysms
Intracranial aneurysms are pathological dilations of blood vessels taking the shape of bulges or balloons mostly on wall sides or at arterial bifurcations. These anomalies bear the risk of rupture and subsequent subarachnoidal hemorrhage, which is associated with high mortality and morbidity rates. Fluid mechanical simulations can yet provide insights into the flow patterns and stress distributions, thus helping the evaluation of aneurysm rupture risks. This work aims at developing a framework for predicting blood clot formation inside intracranial aneurysms and the associated arterial wall remodeling. It should serve as basis to evaluate the effectiveness of a flow diverter stent, and enable its optimization through a Deep Reinforcement Learning workflow. Since the time scales involved far exceeds the ones of current blood flow simulation inside intracranial aneurysms, some techniques, based on Artificial Intelligence for instance, might be used to speed up the predictions.
Pungponhavoan TEP

Acceleration of Digimu simulations through an approach coupling full-field resolution and reduced-order models
Can we predict grain growth more efficiently than ever? My thesis tackles the challenge of significantly enhancing grain growth simulations, moving beyond the slow and resource-intensive methods of traditional PDE-based simulations. The core of my thesis is the development of a new state-of-the-art machine learning framework. This framework is not just about crafting an innovative model or architecture; it’s about constructing a comprehensive dataset that empowers our algorithms to learn, predict, and innovate in ways previously unimagined. We’re stepping into a future where the complexities of grain growth are unraveled through the lens of advanced machine learning technologies.
Eliane YOUNES

AI and digital twins in metallurgy- Front trackin modeling of evolving interface networks.
This thesis focuses on the application of artificial intelligence and digital twins in metallurgy. The objective is to develop computational strategies for designing metallic materials with low environmental impact. The microstructure of materials is crucial for their properties and durability. Understanding and predicting these evolutions is essential for the industry. Multiscale modeling is employed, with a emphasis on mesoscopic scale simulations. A 2D front tracking method called ToRealMotion is explored. Deep neural networks will be used to enhance the efficiency of computational operations and automatically interpret microstructural features.